M. Calzavara, Y. Kuriatnikov, A. Deutschmann-Olek, F. Motzoi, S. Erne, A. Kugi, T. Calarco, J. Schmiedmayer, M. Prüfer, “Optimizing Optical Potentials With Physics-Inspired Learning Algorithms”, Phys. Rev. Appl. 19 (2023). https://doi.org/10.1103/PhysRevApplied.19.044090
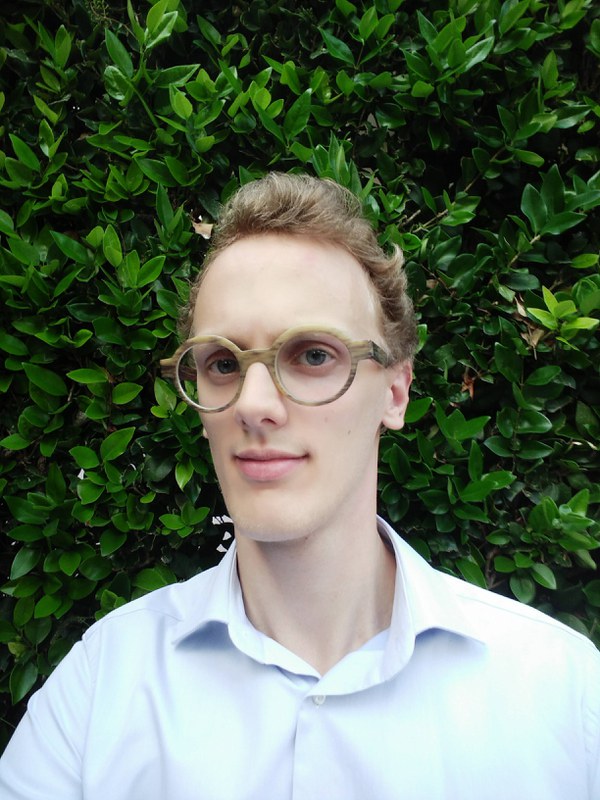
Martino Calzavara
Adresse
Forschungszentrum Jülich GmbH
Wilhelm-Johnen-Straße
52428 Jülich
Peter Grünberg Institut (PGI)
Quantum Control (PGI-8)
Gebäude 05.3 / Raum 275b
About me
I am a PhD student from Padova (Italy), with a long standing ambition of turning theoretical knowledge into practical tools. This, together with my training as a condensed matter physicist, brought me into the field of quantum technologies, and led me to start my PhD at FZJ in 2020. The scope of my research ranges across the quantum software stack. Starting very close to the hardware, I developed methods to control optical dipole potentials in experiments with ultracold gases. Climbing up the abstraction layers, I devised hybrid analytical/numerical methods to optimally transport cold atoms in optical tweezers.
Currently, I am analyzing the optimization landscape of control problems, focusing on data-based predictive models of the latter. The leitmotiv of these projects is using prior physical knowledge to inform optimization and learning, which reduces the data requirements from the experiments.