Assimilation of soil moisture data from a cosmic-ray sensor network in the Rur catchment
Soil moisture is an important driver for water and energy exchange at the land surface and a correct prediction of soil moisture with hydrological models is relevant e.g., in the context of flood forecasting, water management, climate projections and agricultural production. Predictions with hydrological models are always associated with a considerable degree of uncertainty, which can be quantified and reduced through the infusion of observation data by data assimilation techniques like the ensemble Kalman filter. Soil moisture data can be derived from a variety of sensors at different spatial (cm - km) and temporal (minutes - several days) scales ranging from in-situ sensor networks to large-scale remote sensing products. Cosmic-ray probes are a relatively new sensor type which has the advantage that the sensor footprint (approx. 300 meters) and the temporal resolution (hourly to daily) correspond quite well to the typical spatial and temporal resolution of catchment-scale hydrological models. Recently, a network of cosmic-ray probes was established in the Rur catchment in the framework of the TERENO initiative. This observation network currently provides daily averaged soil moisture data at 13 locations within the Rur catchment.
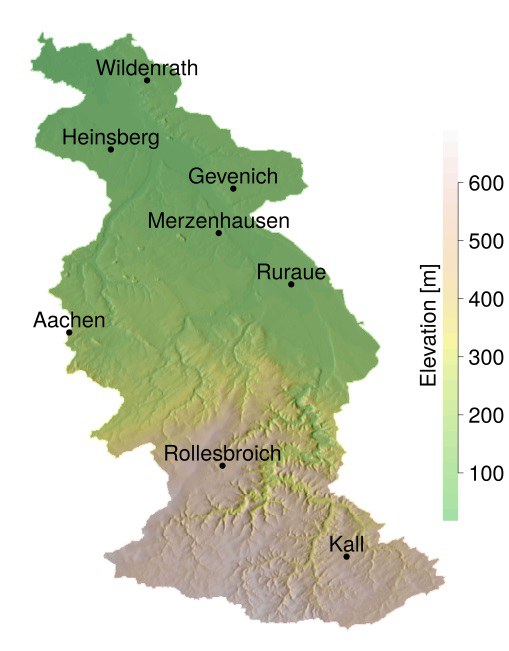
In this study, we performed data assimilation experiments with a TerrSysMP model of the Rur catchment in which the information gain through the assimilation of soil moisture data from this cosmic-ray observation network was evaluated. The TerrSysMP model of the Rur catchment consisted of the land surface (CLM 3.5) and the subsurface part (ParFlow) of TerrSysMP which were driven by hourly meteorological forcing data from the COSMO analysis of the German Weather Service. An ensemble of model realisations was generated by (1) perturbing subsurface hydraulic parameters with spatially-correlated noise and (2) by perturbing atmospheric forcing data (precipitation, longwave/shortwave radiation, 2-m ground temperature) with additive or multiplicative noise. Soil moisture data from the cosmic-ray sensor network were assimilated on a daily basis with the ensemble Kalman filter for the period April – August 2013 where data from eight cosmic-ray stations were available.
Results showed that the daily assimilation of soil moisture data from the cosmic-ray sensor network generally improved the soil moisture prediction of the TerrSysMP forward model by reducing a wet bias for several stations and by enhancing the temporal dynamics. Soil moisture assimilation partly changed the energy flux partitioning at the land surface leading to an increase of sensible heat fluxes and a reduction of latent heat fluxes especially in the northern part of the study region. Additionally, the joint estimation of soil moisture and hydraulic conductivity with the ensemble Kalman filter led to a better soil moisture characterization in simulation periods without available observation data. It was also investigated how the performance of soil moisture assimilation is affected by the conceptualization of the subsurface and it was found that a proper physical description of groundwater dynamics is important for the prediction of soil water dynamics and the effectiveness of soil moisture assimilation.
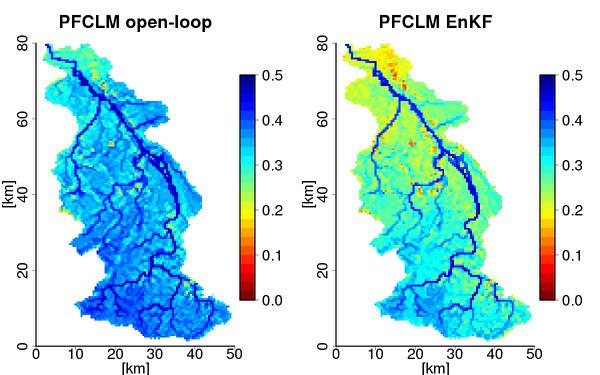