Mechanisms on Neural Information Processing
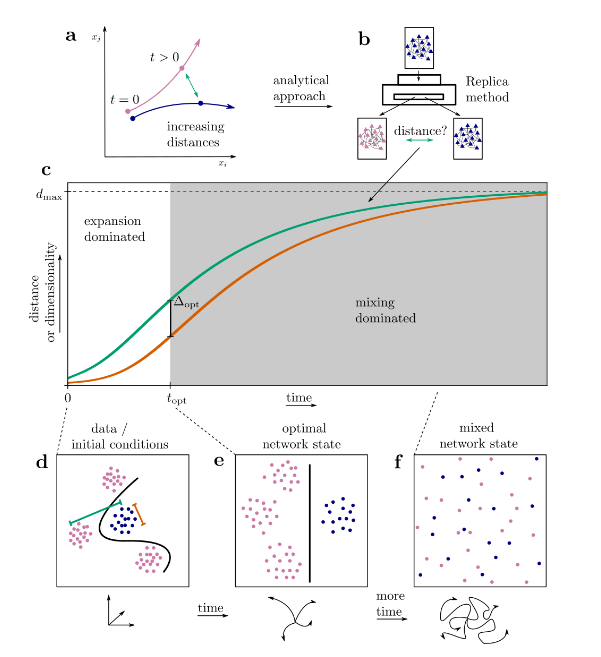
Copyright: Increased computational performance by transient dimensionality expansion of chaotic neural networks (copyright: Keup et al., 2021 (CC-BY license))
Several features render biological information processing distinct from information processing in artificial neuronal networks and makes their study particularly inspiring. The connectivity of brain networks is dominated by recurrence, rather than being primarily feed-forward structures; they exhibit rich temporal dynamics, rather than being static functions of the input; their activity is highly variable or stochastic, rather then being deterministic; neurons communicate with impulses rather than with continuous signals. The aim it to understand the potential for information processing offered by these distinct properties of biological neuronal networks. To this end, we employ insights into the network dynamics and use mathematical techniques to decompose and analyze how recurrent networks transform stimuli into their state and output. Often such equivalent mathematical descriptions have a feed-forward structure so that, on a formal level, they are comparable to artificial neuronal networks. By comparison of biological spiking communication and communication by continuous signals in artificial networks, we could show that discrete communication leads to an increased sensitivity of the network to weak input signals and an accelerated processing of the latter, consistent with the fast processing of sensory stimuli in the brain.