Structural connectome-based predictive modeling of cognitive deficits in treated glioma patients
In glioma patients, tumor growth and subsequent treatments are associated with various types of brain lesions. We hypothesized that cognitive functioning in these patients critically depends on the maintained structural connectivity of multiple brain networks.
The study included 121 glioma patients (median age, 52 years; median Eastern Cooperative Oncology Group performance score 1; CNS-WHO Grade 3 or 4) after multimodal therapy. Cognitive performance was assessed by 10 tests in 5 cognitive domains at a median of 14 months after treatment initiation. Hybrid amino acid PET/MRI using the tracer O-(2-[18F]fluoroethyl)-L-tyrosine, a network-based cortical parcellation, and advanced tractography were used to generate whole-brain fiber count-weighted connectivity matrices. The matrices were applied to a cross-validated machine-learning model to identify predictive fiber connections (edges), critical cortical regions (nodes), and the networks underlying cognitive performance (Fig. 1).
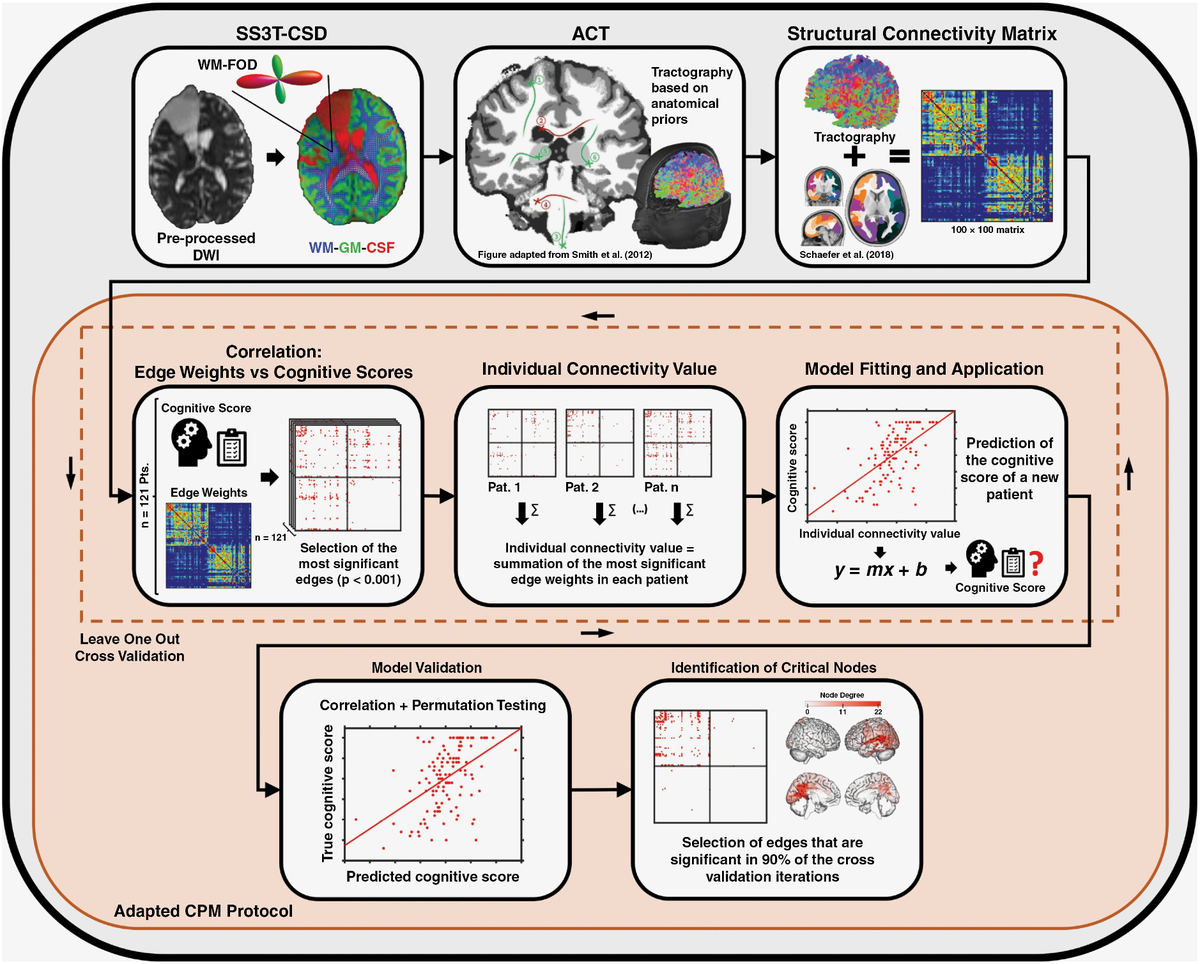
Compared to healthy controls (n = 121), patients’ cognitive scores were significantly lower in 9 cognitive tests. The models predicted the scores of 7/10 tests (median correlation coefficient, 0.47; range, 0.39–0.57) from 0.6% to 5.4% of the matrix entries; 84% of the predictive edges were between nodes of different networks. Critically involved cortical regions (≥10 adjacent edges) included predominantly left-sided nodes of the visual, somatomotor, dorsal/ventral attention, and default mode networks. Highly critical nodes (≥15 edges) included the default mode network’s left temporal and bilateral posterior cingulate cortex (Fig. 2).
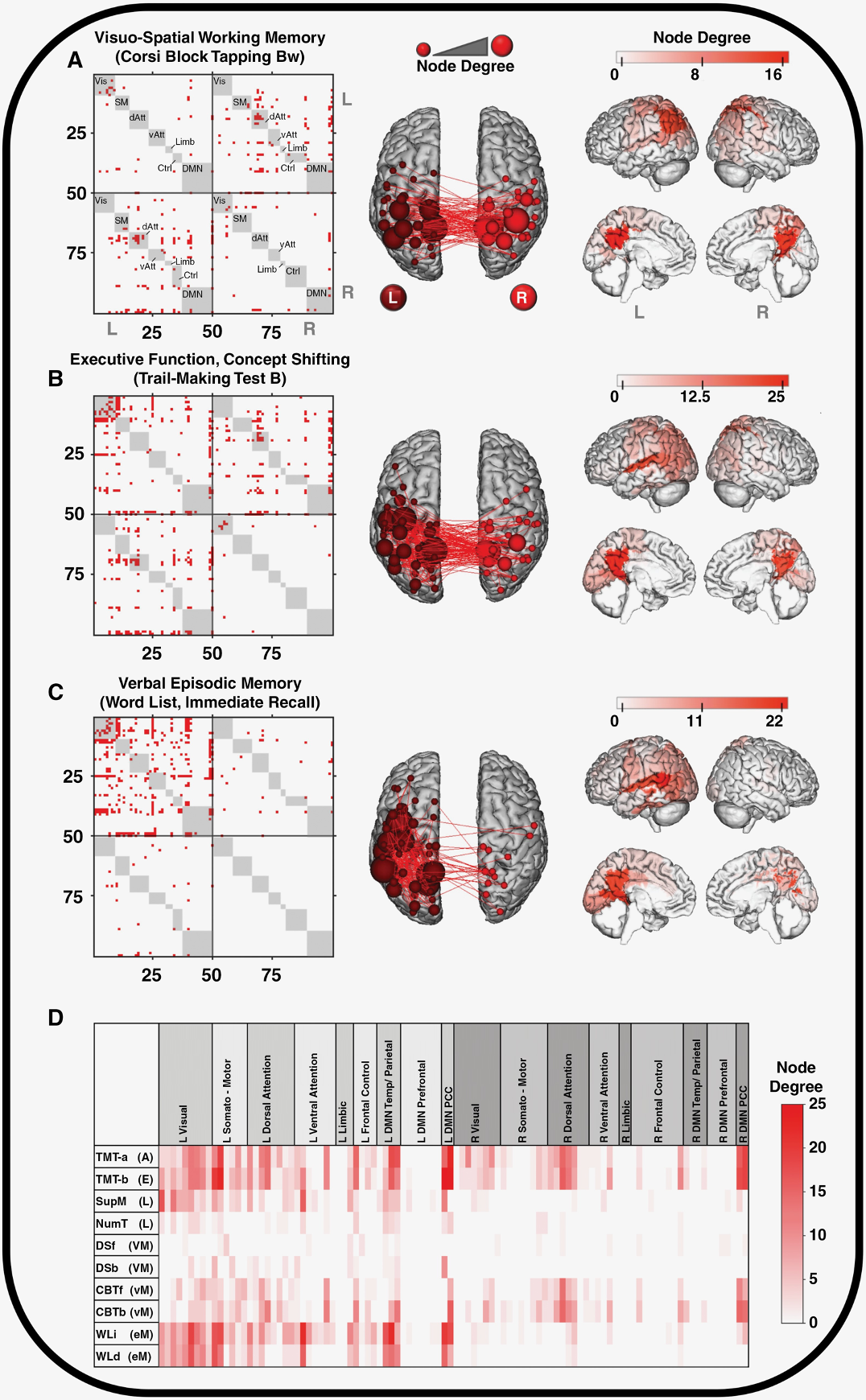
These results suggest that the cognitive performance of pretreated glioma patients is strongly related to structural connectivity between multiple brain networks and depends on the integrity of known network hubs also involved in other neurological disorders.
Publication:
Friedrich, M., Filss, C. P., Lohmann, P., Mottaghy, F. M., Stoffels, G., Weiss Lucas, C., Ruge, M. I., Shah, N. J., Caspers, S., Langen, K.-J., Fink, G. R., Galldiks, N. & Kocher, M. (2024). Structural connectome-based predictive modeling of cognitive deficits in treated glioma patients. Neuro-Oncology Advances, 6(1), vdad15. doi: 10.1093/noajnl/vdad151
Correspondence to: