Machine learning for improvement of upper-tropospheric relative humidity in ERA5 weather model data
Ziming Wang, Luca Bugliaro, Klaus Gierens, Michaela I. Hegglin, Susanne Rohs, Andreas Petzold, Stefan Kaufmann, and Christiane Voigt
Abstract
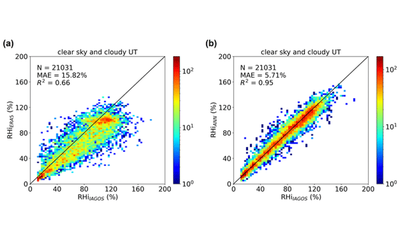
Knowledge of humidity in the upper troposphere and lower stratosphere (UTLS) is of special interest due to its importance for cirrus cloud formation and its climate impact. However, the UTLS water vapor distribution in current weather models is subject to large uncertainties. Here, we develop a dynamic-based humidity correction method using an artificial neural network (ANN) to improve the relative humidity over ice (RHi) in ECMWF numerical weather predictions. The model is trained with time-dependent thermodynamic and dynamical variables from ECMWF ERA5 and humidity measurements from the In-service Aircraft for a Global Observing System (IAGOS). Previous and current atmospheric variables within ±2 ERA5 pressure layers around the IAGOS flight altitude are used for ANN training. RHi, temperature, and geopotential exhibit the highest impact on ANN results, while other dynamical variables are of low to moderate or high importance. The ANN shows excellent performance, and the predicted RHi in the UT has a mean absolute error (MAE) of 5.7 % and a coefficient of determination (R2) of 0.95, which is significantly improved compared to ERA5 RHi (MAE of 15.8 %; R2 of 0.66). The ANN model also improves the prediction skill for all-sky UT/LS and cloudy UTLS and removes the peak at RHi = 100 %. The contrail predictions are in better agreement with Meteosat Second Generation (MSG) observations of ice optical thickness than the results without humidity correction for a contrail cirrus scene over the Atlantic. The ANN method can be applied to other weather models to improve humidity predictions and to support aviation and climate research applications.